Vol. 2 No. 2 (2023)
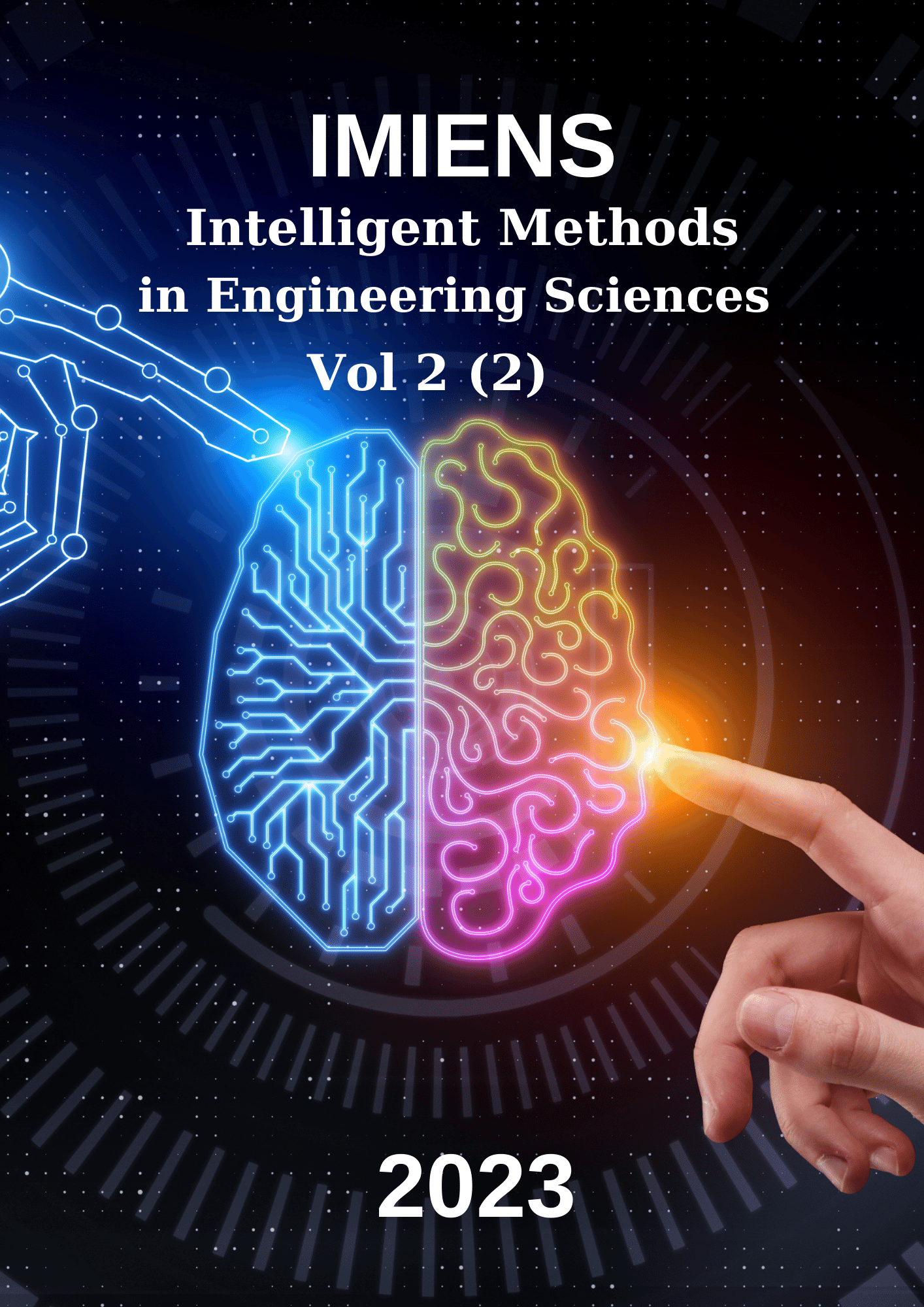
This issue of Intelligent Methods in Engineering Sciences (Vol. 2, No. 2, 2023) explores diverse AI applications including medical diagnostics, robotic vision systems, AR-based rehabilitation, and agricultural product classification. The featured studies demonstrate the practical impact of deep learning and intelligent systems across healthcare, manufacturing, and food technology.
Published:
2023-06-29